How Abnormal Security Leverages NLP to Thwart Cyberattacks
With the emergence of new technology like generative artificial intelligence (Gen AI), cyber threats are growing more sophisticated and more challenging to detect. Traditional security measures often fall short in defending against these advanced attacks. In response, AI is being harnessed for good—utilizing tools like natural language processing (NLP) to revolutionize the cybersecurity landscape.
At Abnormal Security, we harness this technology to provide our customers with robust email protection. By analyzing the vast amounts of text data within emails and other communications, the Abnormal platform can identify subtle signs of malicious intent that might otherwise go unnoticed. Here we’ll dive deeper into how we use NLP to detect small nuances in language and defend against even the most sophisticated threats.
Harnessing the Power of NLP for Threat Detection
NLP plays a pivotal role in modern cybersecurity by enhancing the ability to detect and mitigate sophisticated threats. Abnormal leverages advanced NLP techniques like sentiment analysis and context understanding to uncover phishing attempts, fraudulent communications, and other forms of cyberattacks. Let’s dive into some of these concepts to explain how they work.
Tokenization: The Building Block of Text Understanding
One critical aspect of text understanding is tokenization. Over the past few years, computers have significantly advanced in their ability to understand text. This progress is evident to anyone who has used search engines or AI chatbots. However, it’s important to recognize that computers inherently understand numbers—not text. Therefore, when we input text into a computer, we must represent it numerically.
Tokenization is the process of breaking down text into smaller chunks and assigning numerical values to these chunks. For example, the phrase "click here" can be tokenized as a single phrase, as a list of 11 characters, or as a list of two words. The chosen method of tokenization can significantly impact how the computer processes and understands the text.
If an attacker knows that a system uses word-level tokenization (one token per word), they can exploit this by misspelling words. For example, adding an extra "K" to "click" to form "clickk" can disrupt word-level tokenization but not character-level tokenization. At Abnormal, we employ a defense-in-depth approach by deploying multiple models with different tokenization strategies. For example, we maintain models that use each character-level, subword-level, and phrase-level tokenization. This ensures that if an attacker manipulates input to break one tokenizer, a model using a different tokenizer will still catch the malicious text.

Spotting Malicious Text Signals
Abnormal also utilizes neural networks to analyze all text fields in email messages, including the body, header, attachments, and links. One popular architecture for text models is the transformer, which powers models like BERT, LLaMA, and GPT-4. Transformers connect each word in the text to every other word, allowing them to understand long and complex texts. However, this also makes them slow, due to the numerous connections they need to maintain.
An alternative text model that we use at Abnormal is the Convolutional Neural Network (CNN). This model is extremely efficient at processing short sequences of text. Unlike the transformer, the CNN forces words that are close together in the text, such as words in the same or adjacent sentences, to be relevant to each other. This simple assumption makes the model much faster to run. At Abnormal, we run CNNs on every message we process, which enables us to better spot malicious text and optimally defend our customers.

Adapting Text Models to Evolving Attacker Tactics
Cybercriminals are continually evolving their strategies, often using email text as the initial (but not only) attack vector. For example, in callback phishing messages, attackers urge victims to call a phone number by sending fake purchase confirmations. Attackers may change the brands they impersonate, the structure of fake receipts, the presentation of phone numbers, or the placement of malicious text, but the objective remains the same: to get someone on the phone.

Abnormal combats these tactics by designing our system to run text models on all text extracted from any part of a message—even if the words themselves aren’t inherently malicious. This comprehensive approach ensures there are no structural bottlenecks in recognizing malicious text. Additionally, we automatically retrain our text models with every message processed. Inbound messages, user-reported submissions, and Detection 360 customer reports all contribute to updates in the neural network models. This continuous retraining keeps the models up-to-date with the latest attack patterns, enhancing our ability to protect customers in a dynamic threat landscape. Abnormal also uses advanced AI to parse and analyze text within images and attachments, detecting hidden threats that traditional security measures might miss. This capability enhances the overall security posture by identifying malicious content embedded in various file formats, ensuring comprehensive protection against sophisticated cyberattacks.
An Abnormal Commitment to Customer Protection
In an era where cyber threats are becoming increasingly sophisticated, the role of NLP in cybersecurity cannot be overstated. Ultimately, Abnormal's commitment to leveraging this technology translates to better protection for our customers. The use of multiple tokenization strategies, efficient text analysis models, and adaptive learning processes work together to create a key component of formidable defense against sophisticated cyberattacks. By continuously innovating and refining our techniques, Abnormal stays one step ahead of attackers, providing our customers with peace of mind and robust security.
Interested in learning more about how Abnormal can protect your organization with NLP? Schedule a demo today!
Get AI Protection for Your Human Interactions
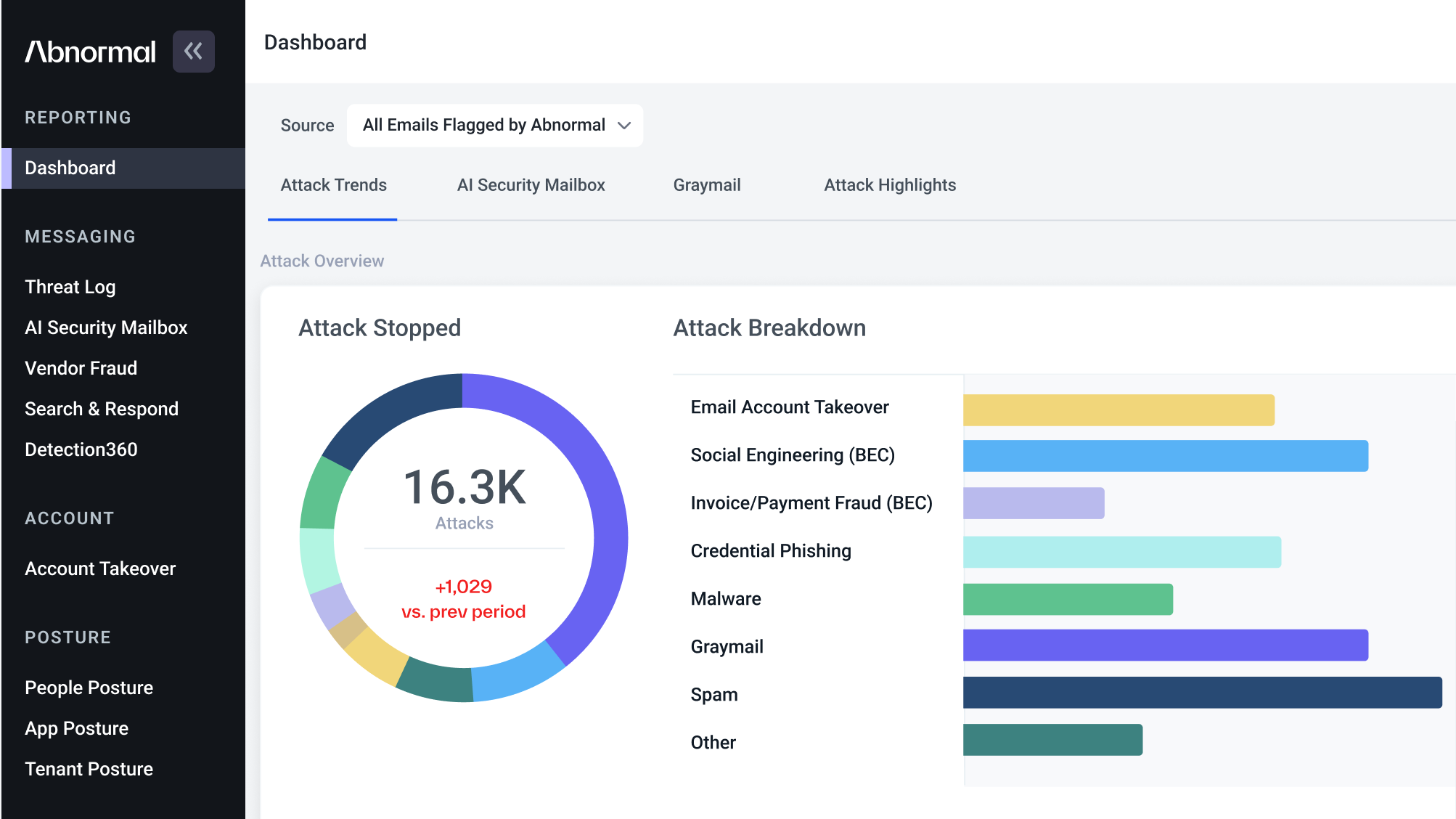