Decisions, Decisions: How AI Makes Determinations and Why It Matters
No doubt, you’ve been bombarded by AI. It seems as if every security solution—every piece of technology at all, really—has an AI component.
When a technological innovation makes it into the public consciousness and completely dominates the mainstream news cycle, it only makes sense that businesses will want to jump on board.
Abnormal Security is an AI-native company, having developed our AI-based email attack detection long before this AI renaissance. The unique API architecture ingests thousands of diverse signals to build a baseline of the known-good behavior of every employee and vendor in an organization based on communication patterns, sign-in events and thousands of other attributes. It then applies advanced AI models including natural language processing (NLP) to detect abnormalities in email behavior that indicate a potential attack.
But the lingering question we often hear in conversations about not only our own AI capabilities but discussing AI in general is: what truly is AI, and how does it work? How does it make decisions? How does it determine when something is actually a threat?
Many security providers label pattern-matching or configuring detection based on static rules as “using AI.” But static rules need to be configured, largely defeating the purpose of AI implementation. And while pattern-matching can be effective, it lacks the ability to make nuanced decisions.
Why Pattern-Matching Isn’t Decision-Making
First, let me undermine my previous paragraph by saying pattern-matching is a component of AI. But when we talk about AI in its current context as a security tool, we’re often talking about deep learning. And pattern-matching is not deep learning.
With pattern-matching, a computer program is fed a variety of patterns and programmed to execute a certain action based on the pattern identified. An example here is a security solution ingesting user activity and being told that is baseline behavior. When an anomalous event occurs, the program executes an action—possibly a notification or remediation action—under the assumption that any anomalous event must be a threat.
While this type of solution can augment a security team by providing insight into unusual behavior, this can lead to excess notification noise and a deluge of false positives. Ultimately, this can cause more harm than good—making it difficult to prioritize investigations and frustrating security practitioners.
Security Tools Need to “Think” Like Security Teams
With deep learning, the computer program is trained to use “common sense.” Rather than throwing up a red flag any time an unusual event occurs and deeming it a threat, deep learning (or an AI neural network) weighs each event. It then correlates these events to determine the likelihood of a threat existing based on the probability of each event occurring in the presence of the others.
Essentially, it is similar to the way humans make decisions: if a drop of water lands on your head, you don’t suddenly assume it’s raining. Maybe it rained the day before, but now the sky is clear. Knowing that, it’s even more unlikely that it’s raining. But if there is an overhang above you, and it’s still damp from the rain the day before, it’s instead likely that a drop of water fell from that overhang onto your head. You’re taking the historical and current inputs and using them to determine the likeliest reason for an event occurring.
Interestingly, deep learning can go a step further still. In this scenario, you could analyze the weekly weather forecast and past weather trends to determine whether (in the face of all of the other signals noted) an anomalous sun shower is probable and that the drop from the overhang was actually a red herring.
While Abnormal deploys a variety of AI techniques and models—from Large Language Models (LLMs) to content analysis to social graphing—deep learning is a major component in determining when a threat is present in a user’s inbox or when a user’s account has been compromised. Abnormal’s data systems are architected to quickly identify anomalies and abnormalities across ingested data sets—in this case, an anomaly is not simply a behavior counter to the established baseline but counter to other anomalies in a given user’s activity.
Let’s take a similar raindrop example but in the context of security and how Abnormal could identify a threat. Say you have a vendor who normally asks for an invoice to be paid at the end of the month but is now suddenly asking for payment in the middle of the month. That may be a threat, but it also may not be. It’s certainly unusual and so pattern-matching may deem it a threat, but it is just as likely that the vendor changed their billing cycle.
What if, though, that same invoice request asks for the payment to go to a different bank account than usual? You’d assume at that point something is amiss, but there is still missing information: what if that vendor changes bank information regularly for compliance or security purposes? Only once it is confirmed through analysis of historical communication data that this vendor never changes banking information can next steps be triggered. In Abnormal’s deep learning models, this is what is meant by detecting the anomalies behind the anomalies—couching each decision in layers of context and analysis to determine with high confidence that a threat is truly present before a decision is made to block an email or, in the case of account compromise, block a user.
Why AI Decision-Making Matters
Why does this matter? Well, aside from detecting more advanced threats and avoiding alert fatigue, AI puts security pros in a better position to investigate threats and frees up time to focus on critical tasks.
Our AI and email security survey of 300 cybersecurity stakeholders found that 94% of participants agree that AI will have a major impact on their security strategy within the next two years.
If a security tool can tell you with confidence that a user’s account has been compromised or a BEC email has landed in a user’s inbox (and, it’s important to mention, that tool can then automatically remediate the issue) a security practitioner will no longer need to address every urgent alert, allowing for more thorough investigation of the most egregious threats and more resources freed up to handle bigger picture security tasks.
In fact, Abnormal saves customers 15+ hours per week normally spent on email threat detection and remediation. What security goals could you accomplish with 15 extra hours each week? If you knew your security tool was making decisions with the speed and precision you expect from your own teammates, what else could you focus on that would otherwise have been cast aside to deal with “urgent” alerts?
Get a demo of Abnormal Security today to learn more about AI-based cloud email security and how it could help you accomplish your security goals.
Get AI Protection for Your Human Interactions
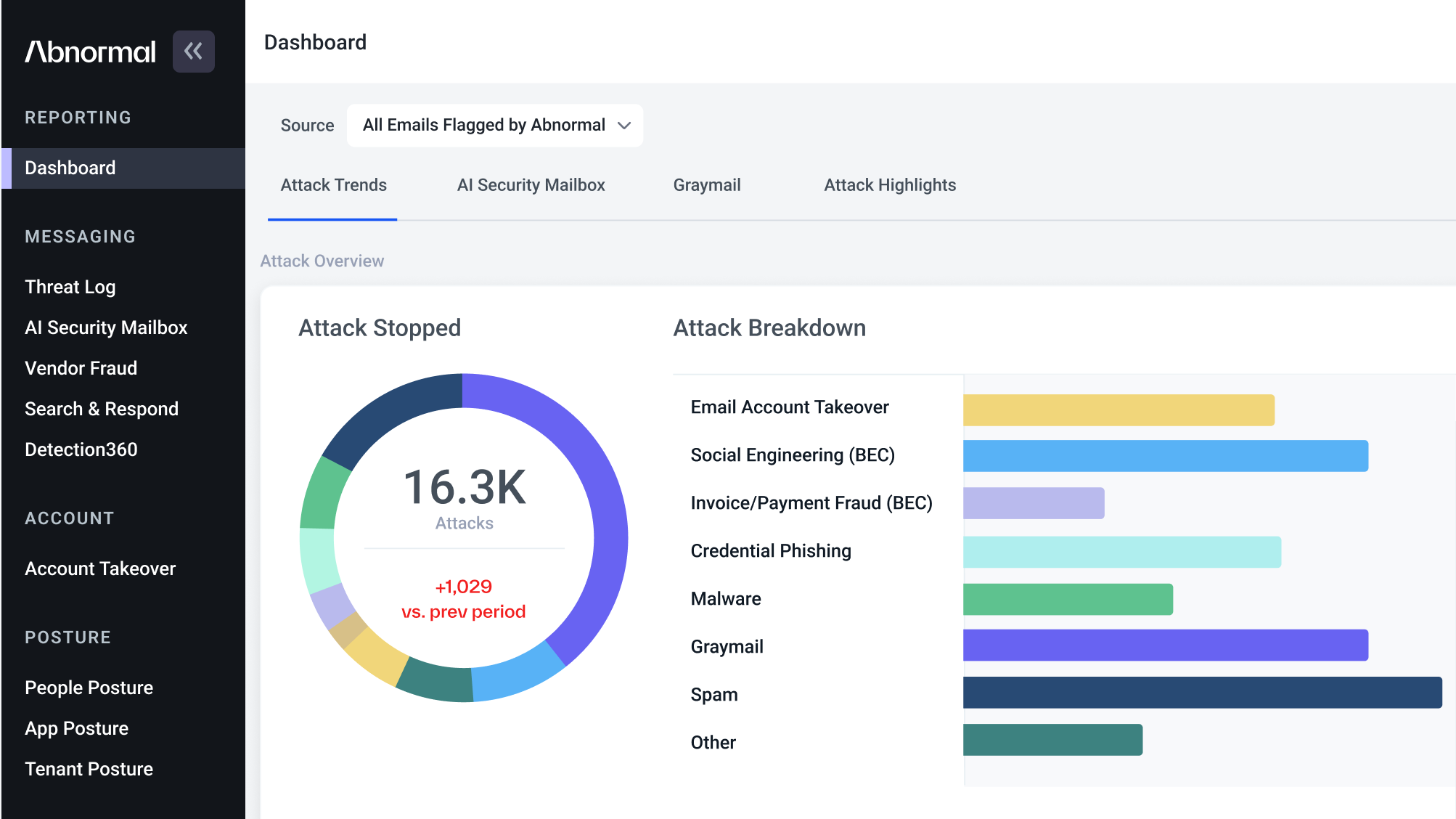