Innovating Email Protection: Writing Detection Rules with LLMs
At Abnormal, our marquee email security product is based on a detection engine. The goal of this engine is to detect cyberattacks among the billions of emails that make up normal business communications.
Our detection engine has many different components, including ML models, attack signature systems, and threat intelligence. One powerful component is our rule engine, which allows us to quickly detect messages with known indicators of compromise. As each message passes through our system, we extract thousands of rich signals describing everything about the message—content, sender, recipient, links, attachments, contextual information, and more.
Our rule engine is a DSL (domain specific language) that can express certain combinations of attributes that should be treated as malicious, such as a bad domain or malicious link. After implementing rules, an email security analyst can then use this rule engine to write detection rules on top of these attributes.
An example rule could be:
( never_seen_sender = true AND from_fqdn_age_in_days < 30 AND attachment_extensions contains "eml" AND body_text_contents contains "urgent_language" )
The rule-writing process is relatively straightforward:
Select a set of attacks that we want to detect.
Study these attacks and craft a rule that matches them.
Validate that the crafted rule matches the selected attacks.
Validate that the rule does not flag any safe messages.
Launch the rule.

Unfortunately, this process can be quite labor-intensive. Hackers develop new attacks at a blistering pace, and we currently see tens of thousands of net-new attacks arise every day. It's simply not feasible for a team of human analysts to keep up. For this reason, Abnormal Security has historically relied upon a machine learning approach in which deep neural networks directly analyze emails.
However, this end-to-end deep learning approach has downsides. For example, deep neural networks are far more difficult to interpret than rules. The growth of large language models and their stunning capability to transform unstructured data to structured data thus raises a question: can we use generative large language models to augment our core machine learning approach with interpretable AI-authored detection rules for better detection?
LLM Rule Generation
Diving into the rule writing process, we see that only step 2, writing a rule from a set of attacks, requires human intervention. Sourcing attacks, verifying that safe messages are not flagged by the rule, and launching the rule can each be fully automated
We can think of step 2 as a translation procedure: the analyst is translating a list of attack messages into the rule DSL. But since large language models like GPT4 are excellent at this kind of translation, we can use a simple one-shot prompt like the following to automate this process:
You are an email security analyst tasked with writing an attack detection rule that flags a set of malicious email messages.
Here is the syntax of your rule engine:
…
Here is the set of malicious email messages that your rule should flag:
…
Your rule:
The output of an LLM fed with this prompt should be a rule that we can test directly. This enables us to craft a rule-writing flow that is completely independent of human intervention.

LLM Rule Generation as Machine Learning
One way to understand what's going on here is to frame this in the language of ML models. The model family is the rule DSL, the training data is the list of attacks, the training algorithm is LLM inference, the hyperparameters are the prompt, and the trained model is the generated rule. An interesting observation that arises from this perspective is that the training data does not contain any safe messages. The algorithm relies on the LLM's prior knowledge of what safe business communication looks like to enable the LLM to write a specific enough rule that flags the target attack messages without flagging any safe messages.
There are several ways to improve this knowledge and, therefore, the generated rule, including adding examples of good rules to the prompt or using a large language model that has been fine-tuned for security applications.
LLM Responsibilities vs. Software Responsibilities
Let's take a step back. One of the core decisions in any LLM-enabled application is the right place to draw the line between the responsibilities of the software system and the responsibilities of the LLM itself. In this framework, we have the following decision points:
Selection of attacks to target
Generation of a rule from these attacks
Evaluation of the rule against the environment of all emails
Interpretation of the evaluation results
Each of these steps could theoretically be powered by a "vanilla" software system or an agentic LLM-enabled system. The system described above only offloads #2 to an LLM, and relies on vanilla software systems to handle numbers 1, 3, and 4.
Intuitively agentic LLM-enabled systems offer flexibility and power at the expense of reliability. This tends to be a poor tradeoff in the domain of evaluation, so steps #3 and #4 are best suited for a vanilla software system. This is especially true at an established organization like Abnormal Security that has invested heavily in rule evaluation infrastructure.
That said, step #1 lends itself much better to LLM involvement, and this is an area of exploration in the future. For example, we could imagine building a system in which one LLM generates attacks and another writes rules to stop them.
Enhancing Defense with LLM-Generated Rules
Rules and heuristics will never be enough to stay on top of attackers. However, LLMs can increase the effectiveness of this strategy by relaxing the human effort bottleneck, while still providing additional controls. The benefit here is that LLM-generated rules can augment a behavioral AI engine—providing defense in depth and additional peace of mind. In addition, we can extrapolate this pattern across domains, as many techniques that are bottlenecked by labor intensiveness today will resurge as LLMs become increasingly popular.
As a fast-growing company, we have lots of interesting engineering challenges to solve, just like this one. If these challenges interest you, and you want to further your growth as an engineer, we’re hiring! Learn more at our careers website.
Get AI Protection for Your Human Interactions
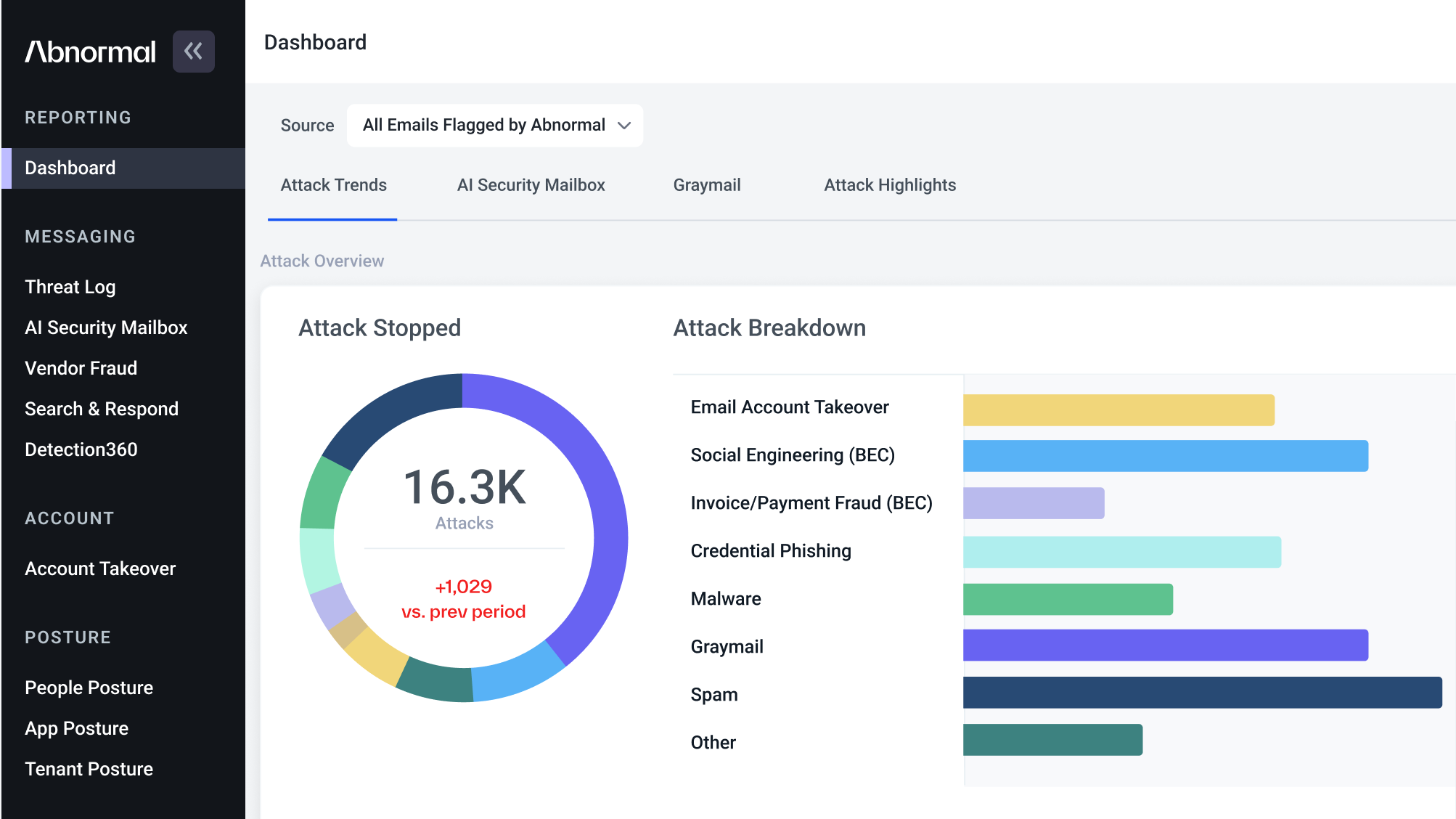