Bypassing SEGs By Abusing Compromised Personal Email Accounts: Real-World Examples
Threat actors are becoming more sophisticated by the minute, using various tactics to bypass traditional security methods such as secure email gateways (SEGs). In a series of recent articles, we have explored some of these tactics in greater detail, including multi-step phishing, OAuth phishing, payment/invoice fraud, and abused open redirects. These are all dangerous threats that, if left undetected, can wreak havoc on an organization.
Today’s installment in the series will explore how attackers can bypass SEGs by abusing compromised personal email accounts. Understanding how cybercriminals are exploiting vulnerabilities in personal email accounts enables organizations to take the necessary measures to secure their systems from further malicious activities. Throughout this article, I will examine the elements of a real-world compromised personal email account attack, and how Abnormal prevented it.
How Compromised Personal Email Accounts Bypass the SEG
In the examples below, we will see messages that touch many, if not all, of these SEG bypass factors:
- Attackers compromise a legitimate personal email account (Gmail, Yahoo, ATT, etc.).
- The compromised account is used to send payload-less malicious emails (text-only).
- Social engineering tactics are used to elicit user engagement, normally leading to financial loss.
Example 1: Barracuda
The first example email originates from a real att.net account.

This email originates from a real att.net account, which does not carry a poor reputation, nor does its sending MTA, as you can see from the MX Toolbox results below:

ATT.net does not have SPF or DMARC records published, so the message cannot effectively be analyzed via Email Authentication.
In examining the body of the message, there are no links or attachments, simply a question about whether the intended recipient orders from Amazon.
The SEG (Barracuda in this case), scored this message pretty low, even with their custom rules invoked, identifying this message as spam based on an empty ‘To’ field and an apparent ‘Reply-To’ mismatch:

So how did Abnormal Security detect and mitigate the risk of this message?

Abnormal Security noticed a number of contextual anomalies including an unusual sender, abnormal recipient pattern, abnormal email signoff, potential personal information theft, and an unusual ‘Reply-To’ domain. These anomalies, when paired with content analysis, made the message a very obvious detection, as highlighted above.
Example 2: Proofpoint
The next example is nearly identical, but bypasses a different SEG and modifies the ‘Reply-To’ to go back to Yahoo, rather than Gmail.

This email originates from a real att.net account, which does not carry a poor reputation, nor does its sending MTA, as you can see in the MX Toolbox results below:

ATT.net does not have SPF or DMARC records published, so the message cannot effectively be analyzed via Email Authentication.

In examining the body of the message, there are no links or attachments, simply a question about whether the intended recipient orders from Amazon.
The SEG (Proofpoint in this case), scored this message pretty low, but agreed with Abnormal’s assessment that the message came from an unknown sender, based on the “unknownsederscore=20” value that is present here:

So how did Abnormal Security detect and mitigate the risk of this message?

Abnormal Security noticed a number of contextual anomalies that, when paired with content analysis, made the message a very obvious detection, as highlighted above.
Example 3: Mimecast
This last example is very similar in practice, but rather than seeking some sort of immediate financial fraud (gift card, fraudulent purchase), the attacker’s eventual goal is not immediately apparent. The message begins by detailing an injury that required surgery.

These messages, when engagement is possible, often lead to disclosure of company policies regarding Insurance, PTO and Sick Leave, FMLA, and other internal process information. Some attackers also seek some sort of payment under the guise of a gift.
This originates from a real AOL account, which does not carry a poor reputation, nor does its sending MTA as seen in the MX Toolbox results below.

AOL does leverage SPF, DKIM, and DMARC, which were all successful in this case, making detection based on email authentication results impossible:

In examining the body of the message, there are no links or attachments, simply a note that someone has taken a fall and required surgery.
The SEG (Mimecast in this case), scored this message pretty low across spam scoring and Impersonation Protect:


A Spam Score of 2 will not trigger relaxed, moderate, or aggressive spam actions from Mimecast.
The Impersonation Protect headers are also interesting, as we see that the Policy used to filter the message is called “Tag Inbound as External” meaning that this policy probably is only tagging messages as external, rather than actively leveraging the condition and “number of hits” settings to actively identify fraud/impersonation.
For those who don’t know, Impersonation Protect is a feature that attempts to detect display name and domain impersonation by identifying various conditions in a potentially-malicious email, requiring a “number of hits” before the message is actioned.
So how did Abnormal Security detect and mitigate the risk of this message?

Abnormal Security noticed a number of contextual anomalies that, when paired with content analysis, made the message a very obvious detection, as highlighted above.
How Abnormal Protects You From Compromised Personal Email Accounts:
Abnormal's innovative platform goes beyond conventional SEGs, providing real-time threat intelligence and actionable insights to security teams.
By analyzing email behavior patterns and context, Abnormal identifies anomalies indicative of zero-day threats, even in the absence of known indicators. Among the three examples above, there are many unique data signals that are used to render high-level summaries. These are triggered by factors such as unusual senders, suspicious body content, unusual financial requests, and more.
Abnormal’s proactive approach leveraging advanced AI and machine learning algorithms ensures comprehensive protection against zero-day threats, bolstering the resilience of organizations in the face of ever-changing cyber risks.
Interested in learning more about how Abnormal can protect you from advanced attacks?
Get AI Protection for Your Human Interactions
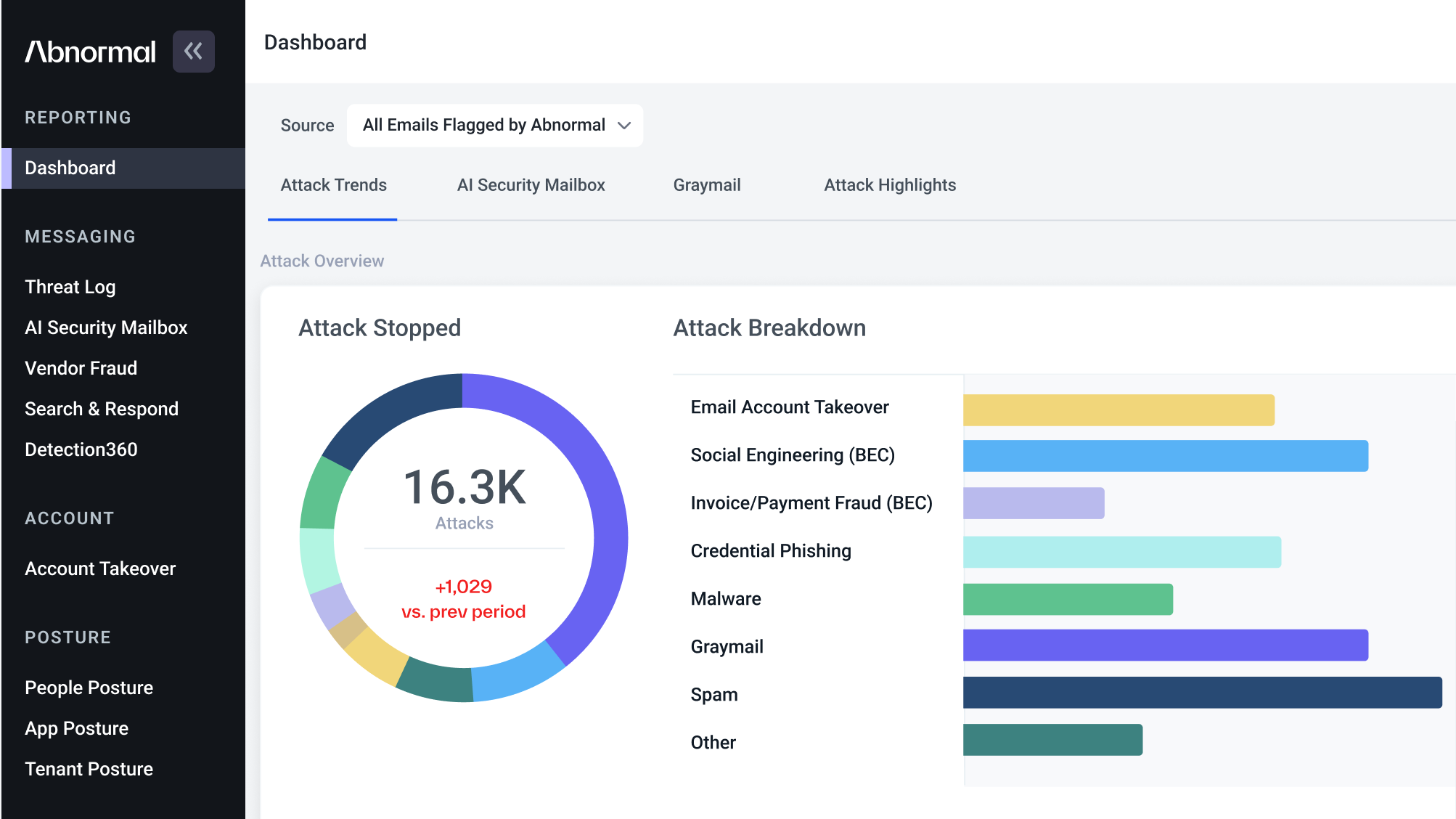