Testing GenAI Products: How Abnormal Ensures Accuracy and Safety
Generative AI, when used correctly, allows companies to build powerful natural language agents leveraging their internal data sources. For conversational use cases, Abnormal Security leverages GenAI in our AI Security Mailbox to provide a virtual expert analyst with context on both Abnormal Security detection intelligence and a customer’s specific security policies.
With our AI Security Mailbox, end users submit suspicious messages to their company’s phishing mailbox. Once Abnormal analyzes the message, we return a personalized report to the end user with the results of our analysis and the judgment of the message. End users can then reply to this initial message with follow-up questions about why we made this judgment and other information about their company’s security policies.

When building these kinds of virtual security experts, accuracy is crucial to end users and the security teams who have enabled our AI Security Mailbox product. Inaccurate responses could lead to confused end users, frustrated escalations to security teams, and, at worst, a user interacting with malicious content.
Conversational AI also has some unique validation challenges, which make it fairly different from typical software testing or even other ML/AI applications. Typical efficacy tests of an ML/AI product might include measuring its precision, recall, or other forms of error rates with respect to ground truth labels. For conversation responses, however, there’s rarely an absolute answer. In fact, there may be several valid responses to a given question.
Keeping Customer Data Secure
Before we discuss accuracy, we first want to guarantee that our conversation agents won’t leak sensitive customer or detection information, either accidentally or through a malicious third party. Since LLMs are statistical models by nature, simply prompting or using exceptional models is not enough to ensure that data will not fall into the wrong hands due to model limitations. In other words, the best and only way to prevent the LLM from leaking information is to not provide it to the LLM in the first place.
At Abnormal, we use a strict limited-access LLM application policy that includes statements such as:
An agent’s abilities and data access scope must be a subset of what the invoking user already has access to.
An agent’s ability to modify an existing resource must be gated by an explicit acknowledgment from the user on the action being taken.
An agent cannot access any external (i.e., non-Abnormal or customer) data sources.
With these policies, our most common GenAI security risks are mitigated. There’s no prompt injection that could exfiltrate data to a third party, no “jailbreak” prompt that could allow a user to access sensitive content they don’t already have access to, and no way for the agent to corrupt or destroy resources without the approval of a human who could perform that action themselves.

Ensuring Accurate Responses
In AI Security Mailbox, a user submits a suspicious email to their phishing mailbox, and they expect a helpful response regarding the results of their submission. In this context, a response being “accurate” can be nuanced and thought of as having all of the following characteristics:
The response is helpful and contains relevant information.
The response is factually accurate.
The response has the appropriate formatting, tone, complexity, and content.
The response adheres to product-specific guidelines and expectations.
We split the evaluation of response quality between two main stages:
Offline Testing - A mix of AI and human review to evaluate the impacts of a specific change to the prompt or agent architecture. This is intended to be thorough yet automated enough to run for all potential changes that could impact the efficacy of the product. A change may undergo several rounds of offline testing before it is actually deployed.
Online Monitoring - Live changes to agent data sources and customer-specific peculiarities could result in subpar customer-received responses. To capture and iterate on these live examples, we use customer reports, sample responses, and AI-identified problematic examples. These then inform prompt improvements and get added to our offline evaluation set.

AI-Based Offline Testing
Our first line of validation relies on the concept of AI-as-a-judge. Given a diverse curated dataset of user questions and customer contexts, we generate responses and use a separate judge LLM, with access to our accuracy rubric, to score them. If the score distribution dramatically changes or degrades, we know to revisit the implementation of the tested change.
To better measure our performance on out-of-domain examples (e.g., users abusing the system, asking off-topic questions, etc.), we also run separate “unrestricted” red team LLMs to pretend to be malicious or unusual users. These conversations are then graded via AI-as-a-judge and used to help validate the quality of a change.
Human-Based Offline Testing
At this point, the change has already been thoroughly tested against our efficacy rubrics. This stage provides additional sanity checks on responses and allows human-in-the-loop validation. If issues are found, we use this information to manually improve our rubric to improve future automated testing.
For certain changes, we also perform manual red teaming exercises to ensure that response accuracy is not critically impacted by the content of data sources (e.g., potentially containing prompt injections) being provided to the agent.
Online Monitoring
Once the change is live, we want to ensure our efficacy is maintained and that the actual responses delivered to customers are accurate.
We source feedback and problematic examples from three different sources:
Direct customer reports
Anonymized sample conversations that are manually verified for quality
A QA-specific LLM, which scans through recent conversations for potential cases of inaccurate or subpar responses and flags these for review
These examples then give us a qualitative and quantitative sense of the quality of responses and how often we generate inaccurate ones. For cases of systematic failure, we use these anonymized examples to help iterate on system improvements and feed into our automated validations.
Launching Safe GenAI Products
LLMs and GenAI are incredible tools for building unique conversation AI products. However, using them to automate previously human-driven processes poses unique risks and challenges. Through strategic safeguards, large-scale GenAI-based validations, and human-in-the-loop verification, Abnormal is able to launch accurate and safe automated analysts to customers. We look forward to continuing to expand our products and capabilities at the intersection of GenAI and cybersecurity.
As a fast-growing company, we have lots of interesting engineering challenges to solve, just like this one. If these challenges interest you, and you want to further your growth as an engineer, we’re hiring! Learn more at our careers website.
Get AI Protection for Your Human Interactions
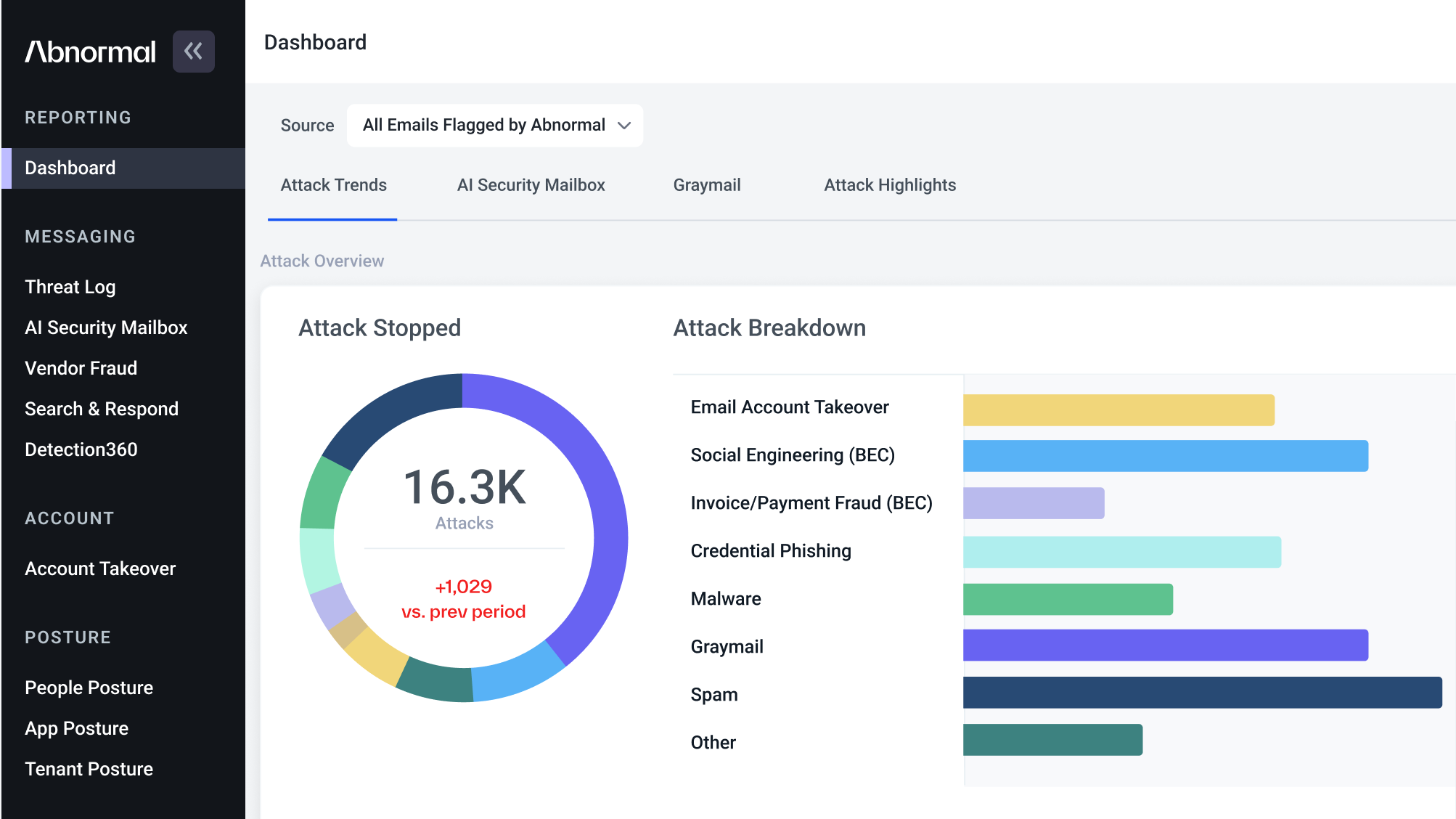