Disciplined and Explainable ML for Email Security
The primary value that Abnormal brings to email security is an advanced, ML-based detection system that can extract and analyze thousands of signals, identify patterns, and adapt over time to detect important attacks–without relying exclusively on threat intel or signature approaches. A common pitfall with ML initiatives in general, though, is a focus on building more and more complex, advanced models and hoping they will magically solve a practical problem.
While Abnormal does spend a lot of time innovating on the model front, the team also has paid special attention to feature engineering—the creation of informative signals from raw data that encapsulate the real problem we are trying to solve. As such, Abnormal produces features and models pertaining to each key part of a message that we may process—ranging from payload analysis (e.g. does the attachment have Javascript?) to business context (e.g. does the body have RFQ language patterns?) to better detect attacks.
Furthermore, we believe it is critical to expose these system internals to our customers to open up “the black box” and give them a real understanding of why Abnormal’s detection system makes the decisions that it does. The rise of new methods in the field of ML explainability (see here and here), in conjunction with this highly intentional design of our system, enables Abnormal to effectively convey deep insights directly in our product.

In particular, we have now introduced an anomaly and behavioral analysis chart on the Details page for every attack, showcasing the different surface areas of messages that we model and the key attributes therein, along with their actual values. In addition to improving confidence in the sophistication and comprehensiveness of the Abnormal detection engine, this visualization has real utility in terms of giving customers insights on specific patterns and risk factors at a more granular level.
Given that ML is the core of the Abnormal approach, we look forward to not only innovating to improve our systems day by day but also to channel these improvements into our product for better customer engagement and understanding.
See what the Abnormal portal could do for you with a free product demo today.
Get AI Protection for Your Human Interactions
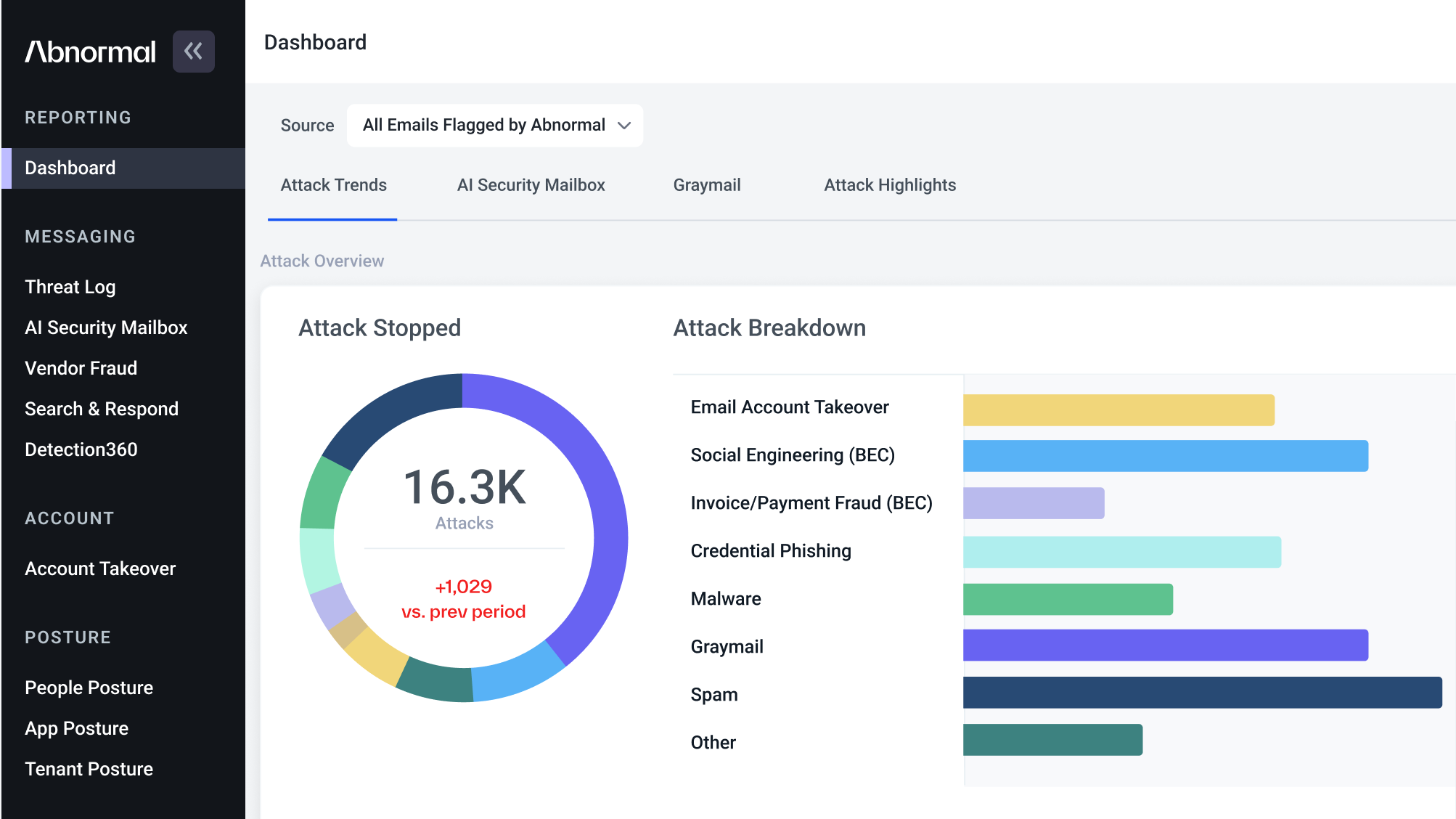