Detecting Email Attacks with Generative LLMs
Cybersecurity is a complex, never-ending battle of wits. As technology advances, so do the methods employed by attackers. In recent times, we've seen a rise in the use of AI for both defensive and offensive purposes. In short, the same technology that helps us stop attacks can also be used to launch them.
We've turned this challenge into an opportunity. We are excited to share that we've integrated with GPT-4, a state-of-the-art large language model (LLM) developed by OpenAI, to enhance our ability to detect sophisticated email attacks.
Security Analysts Tools: Leveraging an AI Copilot for Streamlined Analysis
Our security analysts now use GPT-4 through the secure Azure API as a tool in their arsenal. This has allowed our team to make more confident decisions faster through a ChatGPT-like window that can be used for various open-ended questions.
Depending on what an analyst asks, the GPT-based chat agent can:
Search for similar messages by writing dynamic elastic search queries
Look up suspicious attributes from our vast feature store
Summarize an email’s processing logs or compare it to another email
Interact with our internal databases and scanning tools

Message Labeling: Deep Understanding for Advanced Screening
In some instances, we can use GPT-4 at a near-human-trained level of accuracy to determine if a message is malicious.
Combining more advanced variants of a prompt like "you are a security analyst; label this email" with an internal vector store of labeled messages, we can reliably scan for messages that our primary system falsely detected or missed while providing simple explanations to help identify trends and patch the system. This helps scale our labeling resources and decrease our response latency for misclassifications.

Model Bootstrapping: Using GPT to Boost Existing Models
While GPT-4 can detect novel security tasks with little to no training data, the costs of running such a large model quickly become infeasible for the billions of messages we process every day.
Instead, for our high-volume detectors, we have pipelines to generate synthetic data with GPT-4 that lighter-weight models are trained on. This allows us to rapidly prototype and launch task- or attack-specific models without the need for human labeling, while still being able to serve high-accuracy models at scale.
The Future of Email Security
We believe that the future of email security lies in the intelligent use of AI. We're continuously exploring new ways to harness the power of AI to improve our detection capabilities, and the integration of GPT-4 is just the beginning.
Our vast datasets across attack types allow us to train our own large language models, and we're excited to leverage this to provide the best possible protection for our customers.
We're looking forward to sharing more about how we're using AI to enhance our capabilities in the future. In the meantime, if you want to learn more about how Abnormal detects the most sophisticated attacks, request a demo today.
Interested in learning more about how Abnormal can help your organization protect more, spend less, and secure the future? Schedule a demo today.
Get AI Protection for Your Human Interactions
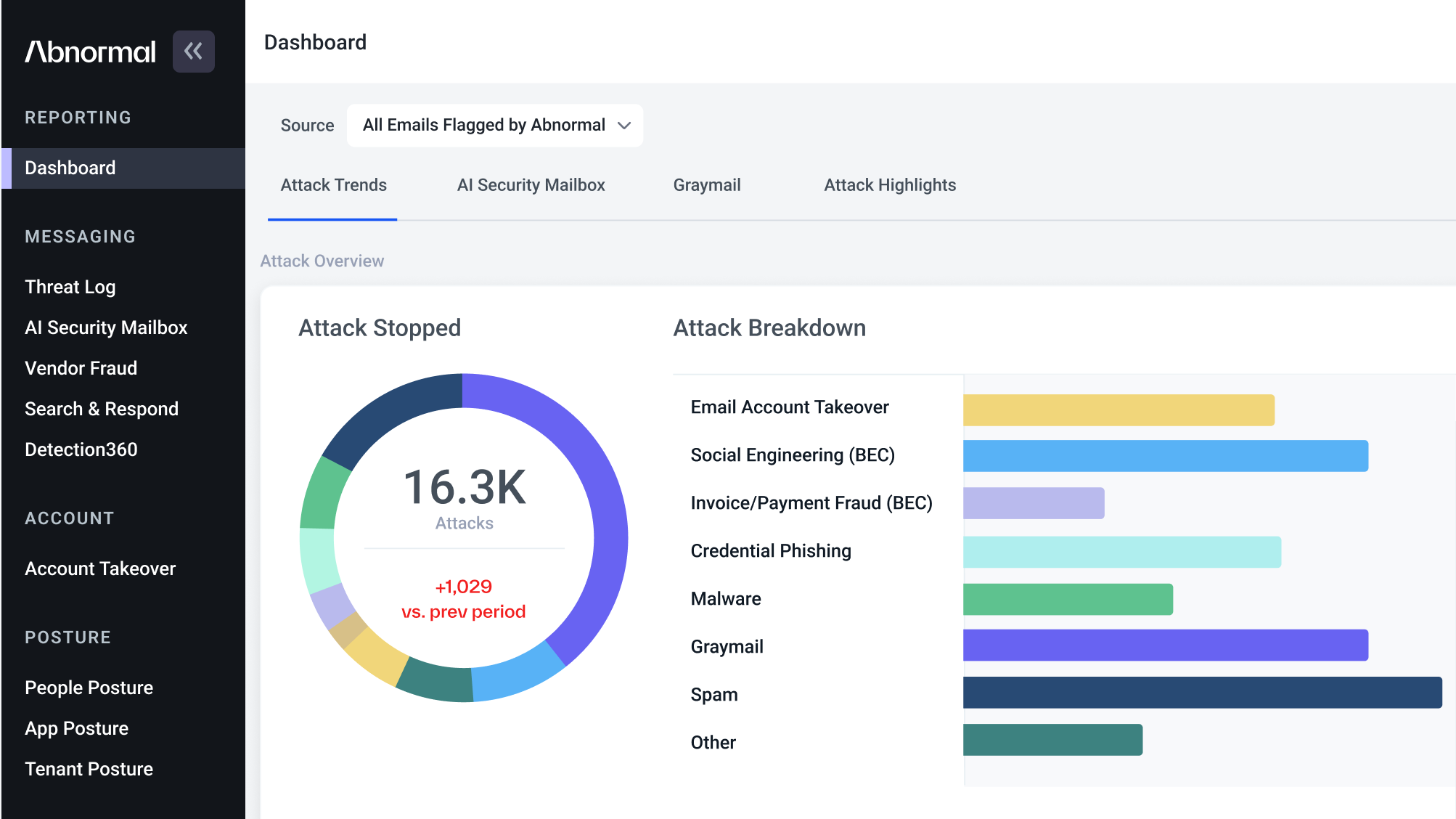