Unmasking Vendor Fraud: Detecting Suspicious Activity in Email Communications
Not all email attacks involve the use of malicious links, malware, or attachments. Increasingly, attackers rely on social engineering tactics to exploit unsuspecting employees. One of the highest value and most pernicious forms of social engineering is vendor fraud.
A typical vendor fraud attack begins with a threat actor sending out counterfeit invoices and redirecting payments to themselves. This is achieved by either impersonating or compromising trusted vendors. Despite being one of the rarest forms of attack we encounter, the potential damage it can wreak is significant.
Detecting vendor fraud is a complex task that requires a multifaceted approach. At a high level, we begin by asking the following questions to determine if an email is likely to be fraudulent:
Is the email related to any financial topic?
Is there anything suspicious about the sender or the message itself?
Are there any suspicious signals with respect to the recipient and sender pairing?
How does this message compare to previous messages from the vendor?
In this article, we’ll discuss how our models are able to analyze every message and establish a baseline of normal behavior to detect anomalies and stop vendor fraud.
Identifying Intent
The initial step in detecting vendor fraud is to identify the intent of the message. Is it an attempt to get an invoice paid? Or is it a ploy to extract sensitive financial information? To distinguish the underlying intent, we employ a comprehensive approach that includes phrase matching, simple text classifiers, and intent modeling with LLM embeddings.
Phrase matching and text classifiers help us establish known patterns of fraudulent requests as well as apply lightweight categorization of messages. LLM embeddings, on the other hand, allow us to have deeper contextual understanding of the message, giving us the ability to categorize never-before-seen text.
This approach equips us with the necessary tools to identify the type of attack we're dealing with. It could be an invoice scam in which the bad actor is attempting to procure payment for an invoice that doesn't correspond to any actual goods or services provided. Or it might be a billing account update scam in which the perpetrator attempts to divert payments to their own account. It could also be wire fraud or any one of the various other forms of financial fraud.
For each of these categories, our models require a different set of signals to detect the attack.
Uncovering Suspicious Message Signals
The next step is looking for suspicious signals in the message itself, which can come in a number of forms.
In vendor fraud attacks, threat actors often make requests that, if fulfilled, will have a significant financial impact—for example, updating billing details or submitting invoices with large dollar amounts. Additionally, these messages typically carry a sense of urgency that is not usually present in standard communications. To capture these subtleties, we employ a variety of text modeling techniques—similar to the intent modeling process.
First, we analyze the sender by studying their email sending patterns and the nature of the emails they deploy. For new senders, we are particularly vigilant about signs of impersonation, such as lookalike domains. Lookalike domains are domains that closely mimic legitimate ones, often by using slight variations in spelling or design. The intent is to deceive recipients into thinking they're interacting with a trusted entity.

Can you tell the difference between the two above URLs? No, they aren’t the same! The first one is our real domain, but in the second URL, the lowercase “l” has been replaced with an uppercase “I”. While it may be hard for a human to discern, these differences are easy for our models to pick up on.
Analyzing Sender and Recipient Patterns
After we've examined the content of the message and scrutinized the sender, our attention then shifts to a more comprehensive analysis of behavioral patterns. This involves leveraging our sophisticated aggregation system, which is capable of tracking and quantifying intricate patterns of behavior. The system takes into account various data points, including:
How often have the sender and recipient communicated?
How often does the recipient receive invoices or other payment-related emails?
When they do receive invoices, are they sent from many smaller vendors utilizing free hosting domains like Gmail? Or are they from more established vendors?
By investigating these elements, our models are able to establish a baseline of normal behavior for each sender and recipient. This enables our models to understand how unusual a given message is within the broader context of the recipient and sender’s past communication patterns.
Looking for Anomalies
Finally, in the case of vendor compromise, we have to detect when an email sent from a trusted vendor’s address was actually sent by an attacker who has compromised the vendor’s account. To determine when this is the case, we scrutinize normal sending behavior patterns from vendors and closely monitor for any deviations.
We monitor a number of signals that we expect to remain constant between two emails from the same sender. These could be something as simple as the time of day an email is sent to more intricate text-based indicators that form a unique digital fingerprint for each sender. When the fingerprints change and coincide with a suspicious request or other red flag, such as an invoice or billing update, it signals a potential threat.
Having gathered these message, sender, and recipient signals, we are now equipped to train our models that form the backbone of our vendor fraud detection strategies.
Stopping Vendor Fraud with Behavioral AI
At Abnormal, we understand that each email communication is unique, with its own context and subtleties, and our models are designed to capture these nuances effectively. By continuously learning and adapting to new patterns of suspicious activity, we can continue to ensure the safety and integrity of your communications in an ever-evolving digital landscape.
Interested in learning more about how Abnormal can help your organization protect more, spend less, and secure the future? Schedule a demo today.
Get AI Protection for Your Human Interactions
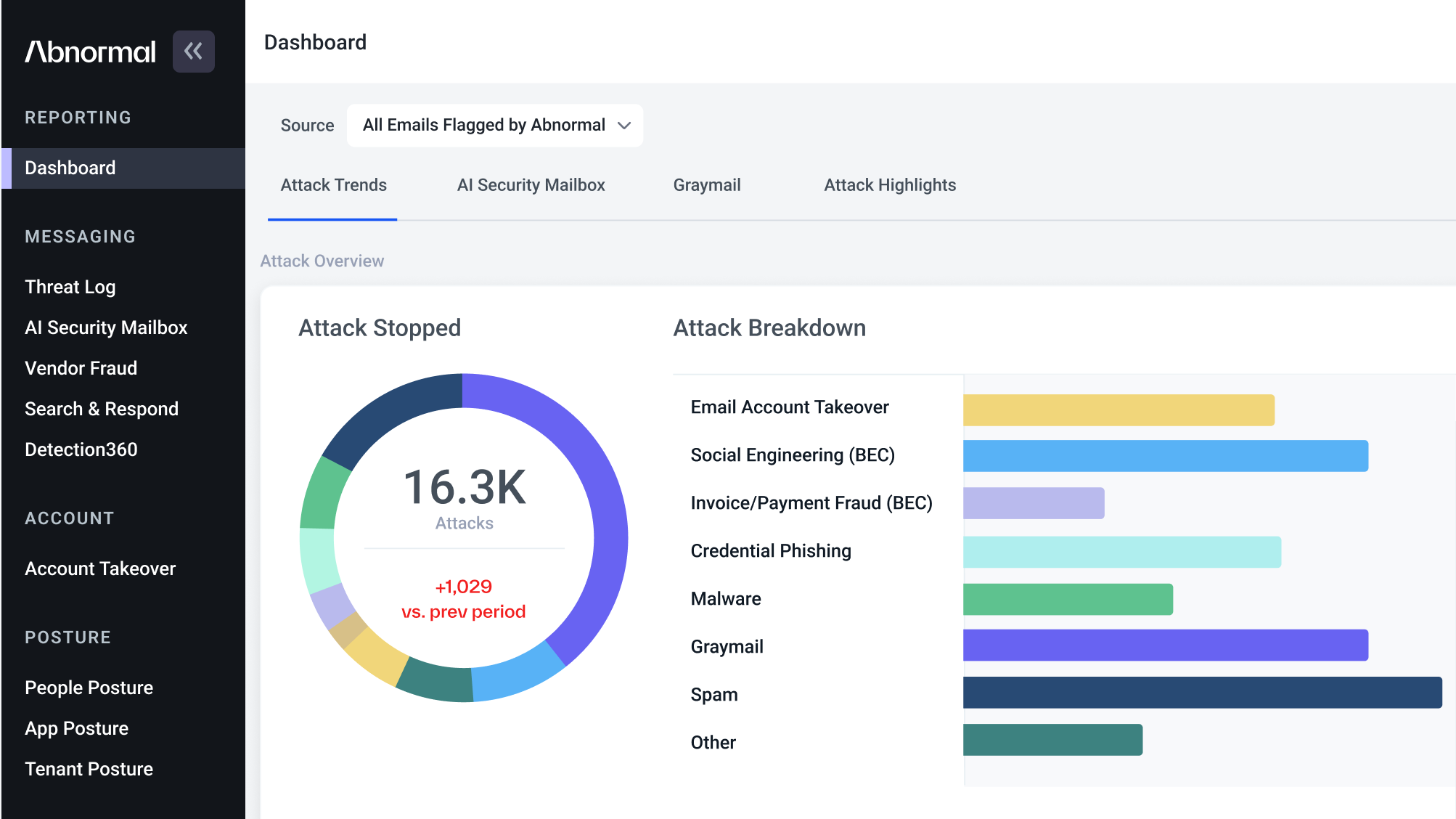