What Is Data Loss Prevention (DLP)?
Data loss prevention (DLP) is a set of software and processes that work together to ensure data is properly used, stored, and protected. It's part of an overall cybersecurity strategy to prevent data breaches and other unauthorized sharing of sensitive data.
DLP is also useful to simplify data archiving and visibility which can help improve productivity. But organizations may also need to implement DLP to stay in compliance with data privacy regulations like HIPAA, PCI-DSS, or GDPR to name a few.
How Does DLP Work?
Data loss prevention solutions use content analysis and a determined set of rules to identify and classify sensitive data, monitoring data movements to detect potential misuse.
Organizations can keep a close eye on access and alteration of confidential data like IP or PII. This helps prevent cybersecurity incidents like employees accidentally or maliciously sending data outside of an organization's network.
One example: a DLP policy can scan large datasets for driver’s license numbers. If this data is detected in a file transfer, the DLP policy can flag it, prevent alteration, encrypt it, or remediate it in other ways.
A DLP strategy has three main tenets:
Identify and classify sensitive data.
Understand the context of this data, including storage and access.
Create a concrete policy that applies to this context.
Implement procedures and software to enact the policy.
How DLP Analyzes Data: Content vs. Context and Methods
DLP solutions use two primary approaches to evaluate data while in transit or at rest:
Content awareness scans data for specific keywords and string matches to determine sensitivity. For example, identifying plain-text PII like payment information or social security numbers. A DLP policy can require encryption for such data.
Contextual analysis examines metadata like headers, format, file size, and other properties to determine sensitivity. It goes beyond words to determine the content of data.
DLP solutions frequently use both approaches to examine documents and protect against data misuse. This helps identify sensitive data in documents or find less-obvious information in databases. Think of it as a package: the context is the box the data comes in, and the content is the data itself.
Multiple techniques are used to analyze data and evaluate if it contains sensitive information which needs protection. Some of these methods include:
Rule-based: Uses specific rules to locate sensitive data such as Social Security numbers or credit card information. This is often the first layer of DLP.
Exact file matching: Creates a hash for each document to ensure it wasn't improperly accessed or altered.
Partial data matching: Used for documents with multiple versions so a DLP solution can track all of them.
Statistical analysis: Uses machine learning to learn and identify sensitive data.
Pre-built categorization: Uses compliance standards to create rules which identify sensitive data.
Once a DLP solution uses analysis to identify sensitive data, it can then trigger policy violations to keep data secure. For example, if an employee tries to email data flagged as sensitive to an unauthorized recipient, a DLP solution can detect the policy violation, and block the email from delivering.
Types of DLP
There are four types of DLP software. While each type has the same objective, they have different methods of preventing data loss.
Email DLP: Email communication often contains sensitive data which DLP can monitor and filter. This can help prevent data leaks, phishing emails, and other social engineering scams.
Network DLP: DLP is implemented on a network to monitor and take action on all outgoing and incoming data from any device connected to it. This allows all connected devices to receive DLP policy enforcement.
Cloud DLP: Companies are frequently transitioning to the cloud, and it needs a DLP solution to protect sensitive data uploaded to it. Cloud DLP can improve visibility and ensure data is secure.
Endpoint DLP: Endpoint refers to devices like servers, computers, or mobile phones. Endpoint DLP protects these devices no matter if they are connected to the company network or not.
Causes and Examples of Data Loss
DLP solutions can prevent a variety of data exposure. Some common causes of data loss include:
External threats: Criminals often use social engineering to trick employees into handing over sensitive information. Criminals may also try to penetrate a cybersecurity system and attempt to install ransomware.
Insider threats: Employees may intentionally send sensitive information outside the network, or criminals will take over an email account and use it to manipulate other people to send confidential information or pay a fake invoice.
Unintentional leaks: Employees may accidentally send private information outside the network or fail to follow security guidelines like encrypting sensitive data.
Implementing a DLP solution is part of a multi-layered cybersecurity approach to preventing the loss of data.
How to Implement DLP
Data loss can occur at any time, so organizations should ensure they are constantly monitoring their network for threats. DLP solutions are a necessary tool to protect data from getting improperly sent out of the network or any other type of unauthorized access.
Depending on an organization's industry and local laws, data privacy compliance is also a reason for implementing a DLP solution.
DLP is not a set-it-and-forget-it process. It takes considerable planning, implementation, and maintenance to create a successful DLP program. According to Gartner, 35% of DLP implementations fail.
When choosing how to prevent data loss an organization may benefit from considering best practices:
Create a DLP strategy: A strategy will help an organization create DLP policies and procedures to implement in a DLP solution. An organization should consider its unique requirements, including any compliance standards. The strategy should also cover the ways data needs to be monitored and protected.
Audit and assess inventory: Organizations need to identify where data is stored, where it's coming and going, and if it contains sensitive data. Part of the process is classifying the risk of data in the event of a breach. This helps a DLP solution determine what rules and policies apply.
Establish evaluation criteria: There are many content analysis techniques deployed by DLP providers. It's useful for organizations to evaluate which techniques are used against a real corpus of sensitive data. This will help determine how accurate a DLP solution is, especially regarding an organization's specific needs.
Train employees on security awareness: Every employee plays a crucial part in protecting data. A security awareness training program can ensure employees understand their role and responsibilities for a company's data security.
Cybersecurity practices also need constant evaluation to ensure there are no gaps in security. Routinely monitoring for issues can help an organization detect problems before a criminal does and exploits them. Implementing a DLP solution is only one factor in ensuring data stays secure.
Abnormal Security can stop socially engineered email attacks that put your organization at risk. Schedule a demo to see how it's done.
Get AI Protection for Your Human Interactions
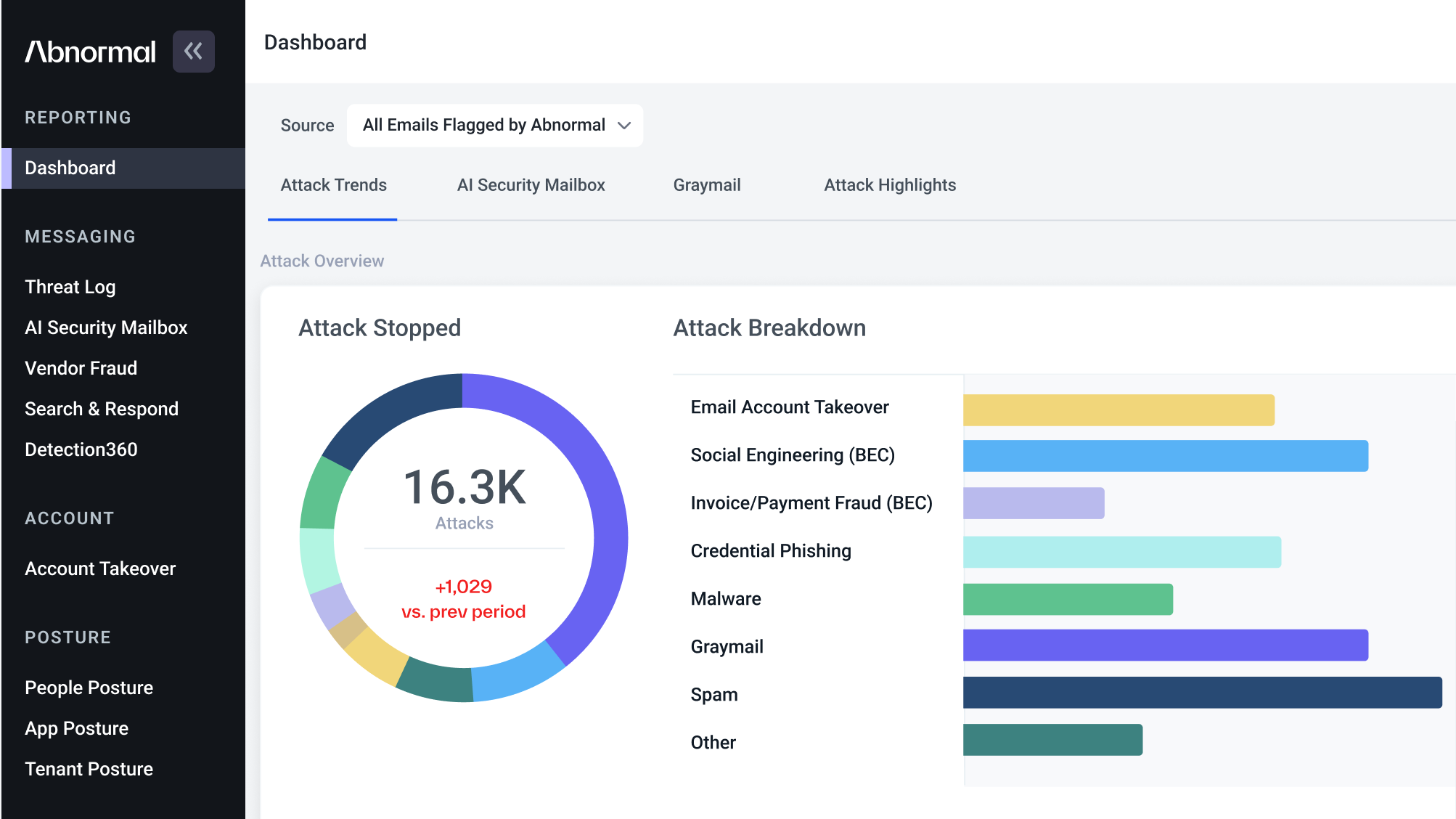